People and culture: your biggest levers for data best practices
Knowing that data organization and hygiene matter in Life Sciences is one thing, but what proactive steps can you take towards achieving a solid baseline? In this Kaleidoscope blog post, we delve into two critical elements: people and culture. If anything here resonates with you, please reach out!
Summary points
- Leadership buy-in and mandate. It's critical for leaders across the org to be aligned on the importance of good data stewardship, and to invest the time and energy into making sure their teams understand and are incentivized to act.
- Communicate, communicate, communicate. Sharing and reiterating the why behind data-related expectations can go a long way in achieving company buy-in. Encouraging dialogue between roles and levels in the org can also build appreciation for any challenges, and help more quickly uncover solutions.
- Self-accountability and bottom-up buy-in. Ultimately, getting individual buy-in is key to building and efficiently maintaining robust data systems. Each person making relatively small, iterative adaptations over time can mean the difference between a continuosly smooth operation, and extremely expensive, time-intensive system overhauls.
In our previous post, we made a case for why being proactive about good data hygiene and organization is mission-critical for anyone working in biotech. Hygiene and organization lay the groundwork for reproducibility and auditability, and are precursors to being able to do exciting things with the data you generate.
Knowing you should be proactive is one thing, but how do you take the next step in becoming proactive? Springing into action is often easier said than done, especially when there isn’t a lot of practical advice out there for scientific leadership and teams on what good data practices look like. Many existing resources are either sales pitches, or tell companies to throw the whole kitchen sink at the problem without taking into account complexity, budgetary constraints, or conflicting priorities.
At Kaleidoscope, we’ve had numerous conversations with a wide range of biotech companies that have had to figure this problem out for themselves, often through a process of trial and error. We’ve condensed and organized the insights from these conversations into suggestions for implementing good data hygiene and organization practices. In this post, we cover perhaps the most important piece of the puzzle: people and culture.
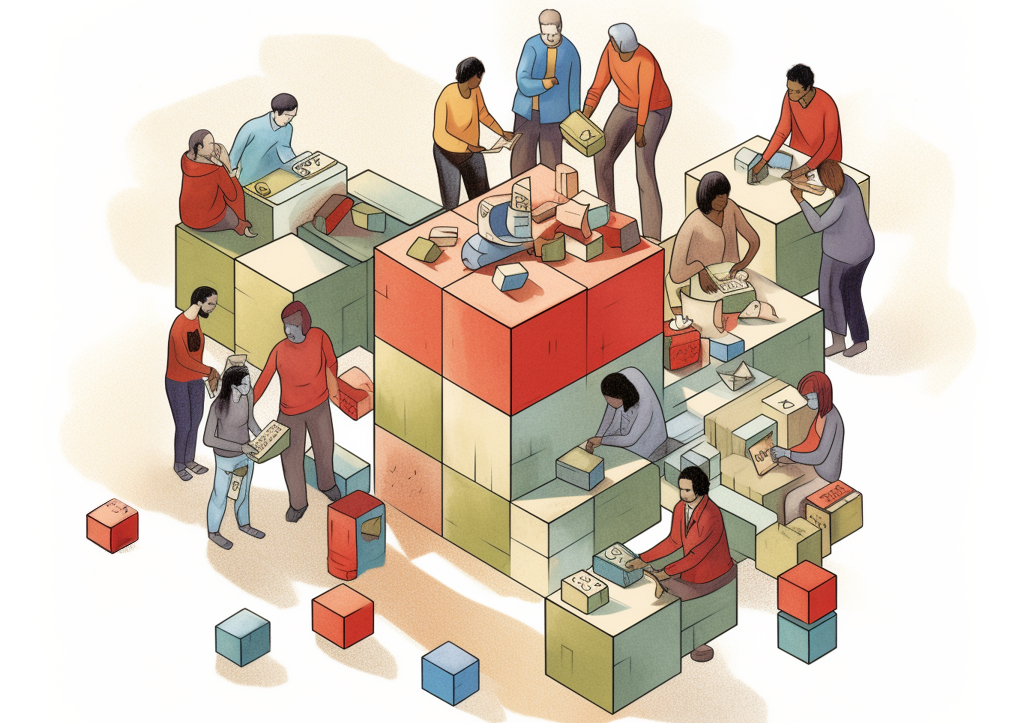
It starts with leadership
Any cultural shift is easier when there’s a leadership team that is convinced and patient – convinced that there’s a problem to be solved, and willing to be patient in getting the solution right.
In biotech, there’s often a tug-of-war between doing things quickly, and building systems that are robust and scalable. For earlier stage companies, the temptation to err on the ‘quick and dirty’ side of the battlefield is natural – as we mentioned in our previous post, science is chaotic, and so much of your time as an early team is consumed by either hair-on-fire problems or the pressure to produce actionable results. The byproduct of this is that data hygiene and organization often slip into an afterthought.
To bring data best practices to the forefront of everyone’s mind, it’s critical for the leadership team to be bought-in. The C-Suite or founding team must be aligned on wanting to build systems that are robust and scalable, and they must be willing to put aside their reservations and invest money and time into getting it right. This percolates to other leaders in the organization too, whether it’s a Head of Biology managing multiple working groups, or a Senior Scientist coordinating a team of three people – it’s critical to be aligned across the organization on what good data practices look like, and the goals you want to achieve by having them in place.
This also means being comfortable with certain hard truths – like the fact that your team might briefly move slower in the immediate-term while they get used to following new data protocols, or the fact that there is no overnight fix. That said, you don’t have to implement a perfect system that will last you ten years, on day one. Rather, it’s about getting the basics in place and starting to build familiarity around organizing, verifying, and cleaning data. Once you’ve done a few reps, you can layer on more complexity as needed.
Leadership influences culture and communication
As a founder or member of the leadership team, culture is one of your biggest levers. Building a muscle around organizing, verifying, and cleaning data is a lot easier if you’ve already created a culture that consistently cares about organization and process in other ways, like in your wet-lab tasks or knowledge base. How organized are your individual-level tools (like electronic lab books)? Do you have a system in place for tracking work that spans people or teams? How logical are your file naming conventions? Being organized across the board makes it easier for your team to see data hygiene and organization as a natural and deeply embedded extension of the way you already do things in other areas of the company.
This culture is mostly crafted by communication. If you’re in a position of leadership, set an expectation of clear communication and lead by example. Make sure people have a shared understanding of why it matters, what the costs of getting it wrong are, and what becomes possible when you get it right. Getting your team to adopt good practices often simply comes down to making sure they understand the why behind the ask.
Communication should also be a two-way conversation – one where you ask your team whether they have adequate support to reach the goals you’re setting for them. Ask them whether they’re well-equipped to track and organize data, and understand where the missing pieces are or where their existing tools are failing them. Clarify who is responsible for what, and co-define what metrics the group should optimize for.
You can also create avenues for conversations between teams. If you have data scientists and engineers working alongside lab scientists, help them communicate to understand the basics of each other’s roles. This might mean, for example, spending a working session sharing what’s challenging about the field. No matter how you go about doing this, the goal should be to build cross-role appreciation over time. Too often, failures in data organization and hygiene come down to scientists not knowing what ‘good’ looks like, or data scientists and engineers not knowing what sometimes makes science chaotic and hard to track.
Communication and accountability is everyone’s responsibility
If you’re a scientist or engineer, it can be easy to shrug off infrastructural problems in data hygiene and organization as someone else’s problem – or, in the more likely case, you may not feel as though your opinion has enough weight to make a difference. However, this usually isn’t true, and acting like an owner and speaking up can go a long way.
For instance, the people picking the software and making the purchasing decisions are often not the people doing the day-to-day work. If there’s a way your work can be easier – like using a new or different tool – you can be proactive in looking for that too, and advocating for it, especially if that tool will enable you to do something important. Sometimes, it might not be a matter of finding a new tool, but advocating for a relatively simple behavioral change. If there’s a leaky bucket in your data management system, your company will want to hear about it and will be grateful that you not only came to them with the bucket, but with ways to plug the holes.
Holding yourself accountable to voicing when there are cracks in your data architecture, searching for solutions, and adopting those solutions goes a long way. The more mature a company becomes, the harder it becomes to retroactively fix poor data hygiene and organization. The cracks begin to harden; there is exponentially more data to clean up and standardize and more employees that have to be trained on unfamiliar processes and tools. This process can consume a lot of time and capital that could’ve been allocated to other things.
Now imagine the alternative scenario: a small team where each employee sees data management as their own responsibility, where they proactively improve processes as and when they fall short. Because this company is still young, improving these processes is just a matter of internalizing relatively small, low-cost changes rather than completely overhauling the existing infrastructure and retraining the organization. It’s better to catch mistakes before they become baked into the way you do things and while you’re still agile – and it’s a lot easier to catch mistakes when everyone sees it as their responsibility.
Keep culture intact by hiring people who care
As your company grows, you should make it a priority to hire people who care about good data hygiene and organization and want to preserve a culture that does the same. This means things like hiring people who straddle the line between software and bio, or who have a history of inspiring good code, data practices, or communication.
For non-technical founders, it’s not as hard as you might think to figure out who cares about good hygiene and organization and who doesn’t. When interviewing scientists, you can talk through some examples of their past work – whether it’s code, notes, or summaries – and discuss why they did or did not organize things a certain way. It’ll quickly become apparent how they approach this topic of well-organized data.
Conclusion
Ultimately, when it comes to getting data organization and data hygiene right, people are the most important part of the puzzle. Regardless of whether you’re a founder, part of the management team, engineer, or scientist, you have a role to play in rallying the company around the importance of good data management and creating a culture that actually cares about it. Often this means communication – about what’s going right, what’s going wrong, how to fix it, what tools to use, or who should be responsible – plus accountability in having those conversations and committing to testing new solutions.
In our next post, we cover the practical steps you can take to implement better data hygiene and data organization.
If you want to chat more about anything we wrote, or you’re interested in finding a way to work together, let us know!