Your moat is your data, not your models
In biotech, your technical moat is key. But not all technical components are equally defensible. In this Kaleidoscope blog post, we make the case for why data - rather than models - are most critical for a defensible moat. As always, if anything here resonates, please reach out.
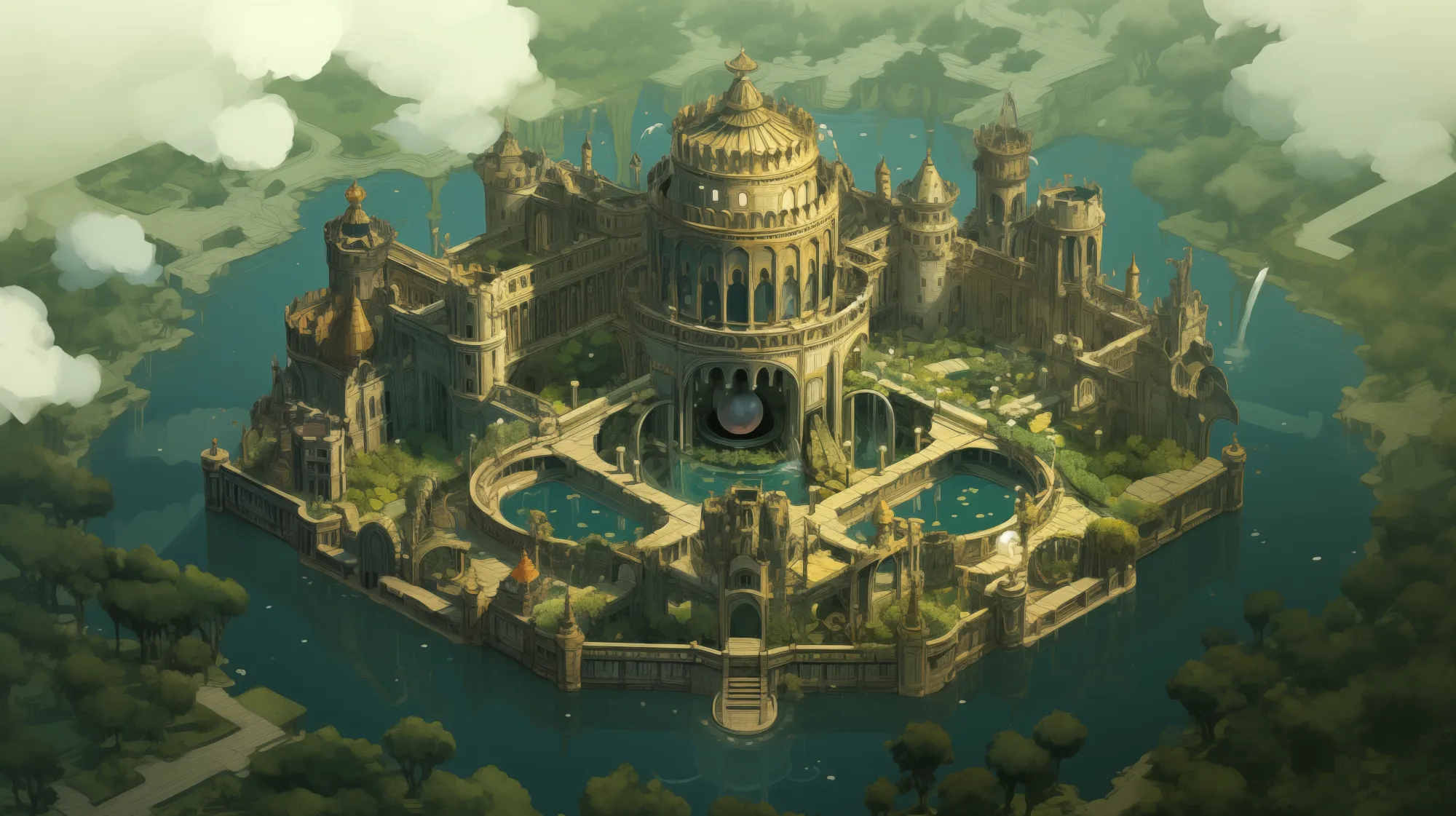
A few weeks ago, we published an article asking therapeutics investors what milestones they look for when they meet with companies that are fundraising. Throughout that piece, it became clear that having a proprietary or defensible data moat is incredibly important, regardless of the stage or size of the company. And, at a time when it seems like everyone is writing about LLMs and AI in bio, it’s interesting that none of the investors highlighted models as an example of a proprietary moat they wanted to see.
Admittedly, we spoke with a very select group of people. Yet, the idea that defensibility for bio companies could hinge more on access to data than on data models, has logic. The development of AI is moving so fast that it feels like we’re standing on dunes of sand that never stop shifting. In the past week alone, Stability introduced Stable Video Diffusion (SVD), a set of AI models for generating video clips from still images; Microsoft released Azure AI Speech to generate photorealistic avatars and replicated voices; Meta launched two new AI-based video editing tools; OpenAI rival Anthropic gave its chatbot an update that allows it to process over 200,000 tokens at once, making it more powerful than GPT-4 – and that’s just the tip of the iceberg.
These updates are exciting and, as Jesse Johnson wrote, there’s no shortage of ideas for how to make more complex and potentially more powerful models. Making these models is becoming somewhat commoditized and, as more models are released, fine-tuned, and updated, they’re making other ideas that once seemed defensible, obsolete. We’ve seen this happen, across multiple industries, to liteweight ‘wrapper’ use cases that were built on top of foundational models. “ChatGPT for X” companies were destabilized by OpenAI plugins, “Chat your PDF” companies were weakened by a ChatGPT update that let users upload PDFs. These startups were in the line of fire of OpenAI and other platforms who owned models that could absorb startups’ current features and future roadmap. This isn’t to say defensibility can’t exist for startups building on top of a foundational model — it’s just a lot harder to achieve.
For companies that are developing their own proprietary models, defensibility can also become an issue. In order for something to be defensible, it has to be differentiated in a way that is difficult to replicate – and there is a computational limit to how successfully you can develop models that significantly outperform others when you feed them all the same information. This concept – that a model can only do the best with the data that it’s been given – is part of the information-theoretic limit. With the same data, the benefits of one model over the other are bound to be marginal – and small, marginal benefits don’t make for a great moat.
So if defensibility is hard to establish when building on top of foundational models, and hard to establish when building novel models, where does it come from?
From the data that is fed into these models. Every model requires data to train with and then more data to fine-tune, and the more relevant data you have, the better your model will perform. AlphaFold is a great example of this in action.
By predicting the 3D structures of almost every known protein from their amino acid sequences, and then making these predictions accessible, AlphaFold was a huge step forward in the bio world. What allowed AlphaFold to use ML to predict these protein structures was the abundance of high fidelity, open data sets to train the model on. Specifically, it leaned on data from the Protein Data Bank (PDB), Uniprot, and metagenomics data from MGnify. PDB is a data set of almost 175,000 experimentally-determined 3D protein structures, UniProt is a protein sequence database with over 220 million protein sequences that are largely annotated, and MGnify is a database with 3 billion non-redundant protein sequences. John Jumper, AlphaFold’s team lead, said these datasets were “essential” in developing AlphaFold. For example, without UniProt annotations, the team wouldn’t have been able to establish the link between AlphaFold confidence and protein disorder.
If these public datasets didn’t exist, there simply wouldn’t be an AlphaFold. Now, imagine if the datasets belonged to a private company — that would be an incredibly strong moat and would give that player the exclusivity to build their own technology that predicted protein structures. Although this is an extreme example, it emphasizes just how critical data is to the whole picture.
If a startup can generate (or somehow has unique access to) the data needed to train and fine-tune models, they hold the keys to the castle more than the people making the models – and, as per our previous article, it seems therapeutics investors are very aware of this, too. To truly create a moat though, data generation may not be enough. For that data to be valuable, it has to be accessible and systematically structured and labeled in a format that can be easily and effectively processed by models or ML techniques. This idea of enhancing the value of data is a large part of why we started Kaleidoscope — to build infrastructure that helps companies manage and track relationships between key decisions, results, metadata, and experimental artifacts, across teams and tools. After all, the best way to ensure you have proprietary access to a dataset needed to train a model is to generate it yourself and keep it well organized.
If you’re interested in finding out how Kaleidoscope can help you with staying on top of your most critical data and decision streams, book a demo and let us show you!