The psychology behind documentation burden in science
We all know scientists hate documenting things. What's behind this and how can we begin to address it, beyond just telling people to "be better at documentation"? Read on in this Kaleidoscope blog! And if anything here resonates, please reach out!
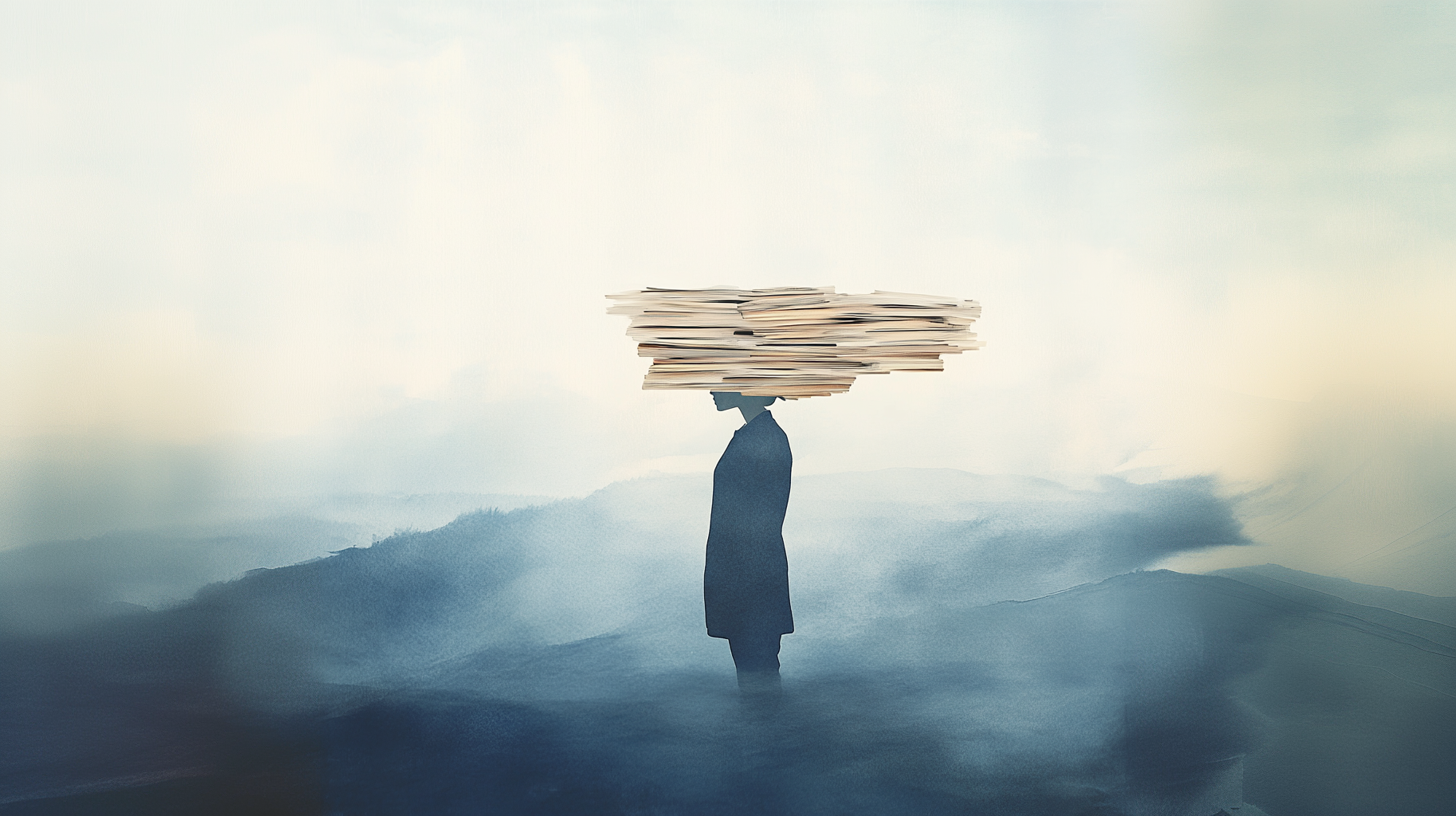
Scientists hate documenting things. It’s one of the most accurate cliches, and we all know about the knock-on effects that bad or no documentation has on reproducibility. We’ve seen a lot less discourse, however, on the psychology behind why documentation feels like such a burden in the first place. Why is there an aversion to it, and why are we so puzzled that, given the existing tools for scientists, it isn’t a priority?
First, the ‘documentation burden’ isn't unique to science. In software engineering, programmers share a similar loathing. On one forum, a frustrated developer asked, "What's the aversion to documentation in the industry?" The responses ranged from "Because experience has proven that no-one reads the docs," to "it is not fun," and - our personal favorite - "Because we know what we're doing! Why should we take time out of the day to write down what we already know and will never forget!?!?"
The problem is, you may know what you’re doing - but you probably will forget things. When you're deeply familiar with a process, it's easy to overlook details that might be crucial for someone else. It's easy to forget the things that you didn't know, or that used to be hard or complicated when you started. Also, perspective is subjective. Documentation that might feel ‘complete’ to you might be impenetrable for the next person; it can be a struggle to put ourselves in the shoes of future readers who might lack our current context and knowledge.
Although engineers aren’t a fan of documentation either, this is something they tend to do much better than scientists. When you’re designing software that’s intended to be used for multiple people, you keep in mind all the types of potential people that might interact with that software. You map out user personas, ask if there are generational differences, vertical differences, geographical differences, and build around them. And you make sure your documentation is robust and accessible to these various permutations of contexts. The same should apply in science. It should be as easy for someone inside your lab as it is for someone outside of it to read your documentation and understand what’s going on.
In science, the difficulty of documentation is amplified by the complexity and variability of experiments. Unlike something like building a car, where processes can be standardized and locked down, experiments require adaptability. Each tweak to the process can affect the results, making comprehensive documentation both crucial and incredibly challenging. When you’re already under intense time pressure - to take a drug to market before competitors, or gathering enough data to hit your next R&D milestones - documentation is bumped down to the status of a luxury you can't afford. It's easy to convince yourself that you'll come back and document everything later when you have more time, but it’s a promise that's rarely kept – mostly because ‘more time’ never comes or, if it does, other tasks that seem more pressing to the immediate future of the company take priority.
Anecdotally, it might also be the case that documentation isn’t taught in academia because of misaligned incentives. When you’re building a biotech company, the purpose of the business is to be able to get a product to market and/or scale a ‘result’ repeatedly. That’s not necessarily the purpose of academia, where you’re more incentivised to show an exciting result and be the first to discover it, than to easily allow others to reproduce it. This is shown time and time again: entire research projects are funded off of data in scientific publications, without anyone really knowing where that data came from or what the exact methods used were.
The purpose of this article isn’t to get into the reasons why documentation is important – the impacts of not doing it are as profound and far-reaching as they are obvious and predictable. If critical parts of important research can’t be understood or replicated, if iteration and collaboration is slowed, or if errors worm their way into work undetected, we all suffer. But when we break down all the above potential reasons behind why scientists don’t like documentation, it appears it’s less to do with behavior and a lack of discipline, and more to do with other themes like:
- The bar for 'good': it’s often unclear what good documentation looks like for different ‘users’
- Cognitive overload coupled with tool inadequacy: documentation adds extra steps to the workflow and absorbs too much time that could be spent on company-accelerating tasks
- Incentives: scientists aren’t incentivized to document their processes
- Time-horizons coupled with uncertainty: documenting a single experiment you're doing today that might be relevant in 2 years makes it really easy to discount the present day value. This is even more acute when you account for dead ends – if you think there's a 90% chance your experiment is irrelevant to "that critical finding", it'll affect your approach to documenting it
With this in mind, perhaps the solution lies not in forcing scientists to change their nature, but in changing how we approach documentation itself. Remember, the goal of a scientist is to research. Anything used in pursuit of that goal are merely tools or a means to an end. So, scientific software should fade into the background and gently guide users to make better decisions around documentation without burdening their work – but, unfortunately, many fail that test. So many tools that promise to help with documentation are too heavy-weight, or only add more steps to the scientific workflow, disincentivizing use.
This is a problem we think a lot about at Kaleidoscope. Fundamentally, to ease the documentation burden, we need to make the process of documenting the most pressing information more intuitive. This is what led us to build our suite of dashboard and ‘data-room’ features – as a way to provide a single, central location where all critical experimental data - and how it relates to project milestones and stakeholder objectives - is easily accessible and synced to the tools that scientists are already using daily. This transparency not only makes it easier for scientists to document their work but also serves as a framework for better documentation practices. It distills the high volume jumble of ongoing experiments into a clearer picture of “this is ultimately what we care most about and have to be able to reproduce.”
So yes, scientists hate documentation. But the good news is, it’s possible to reverse the tide with well-designed tools that address the psychology behind the documentation burden. It's a delicate balance of workflow design and incentives. And, as we continue to generate mountains of experimental data, finding this balance is becoming increasingly pressing.
If you want to chat more about anything we wrote, or you’re interested in finding a way to work together, let us know!