Stop Asking If You're an "AI Biotech Company"
The question of whether a biotech is an AI company or not has created a dichotomy that might be hurting progress in the field. In this post, we explore why the lines between AI and not-AI are blurrier than they seem, and why this is actually a good thing. If anything here resonates, please reach out!
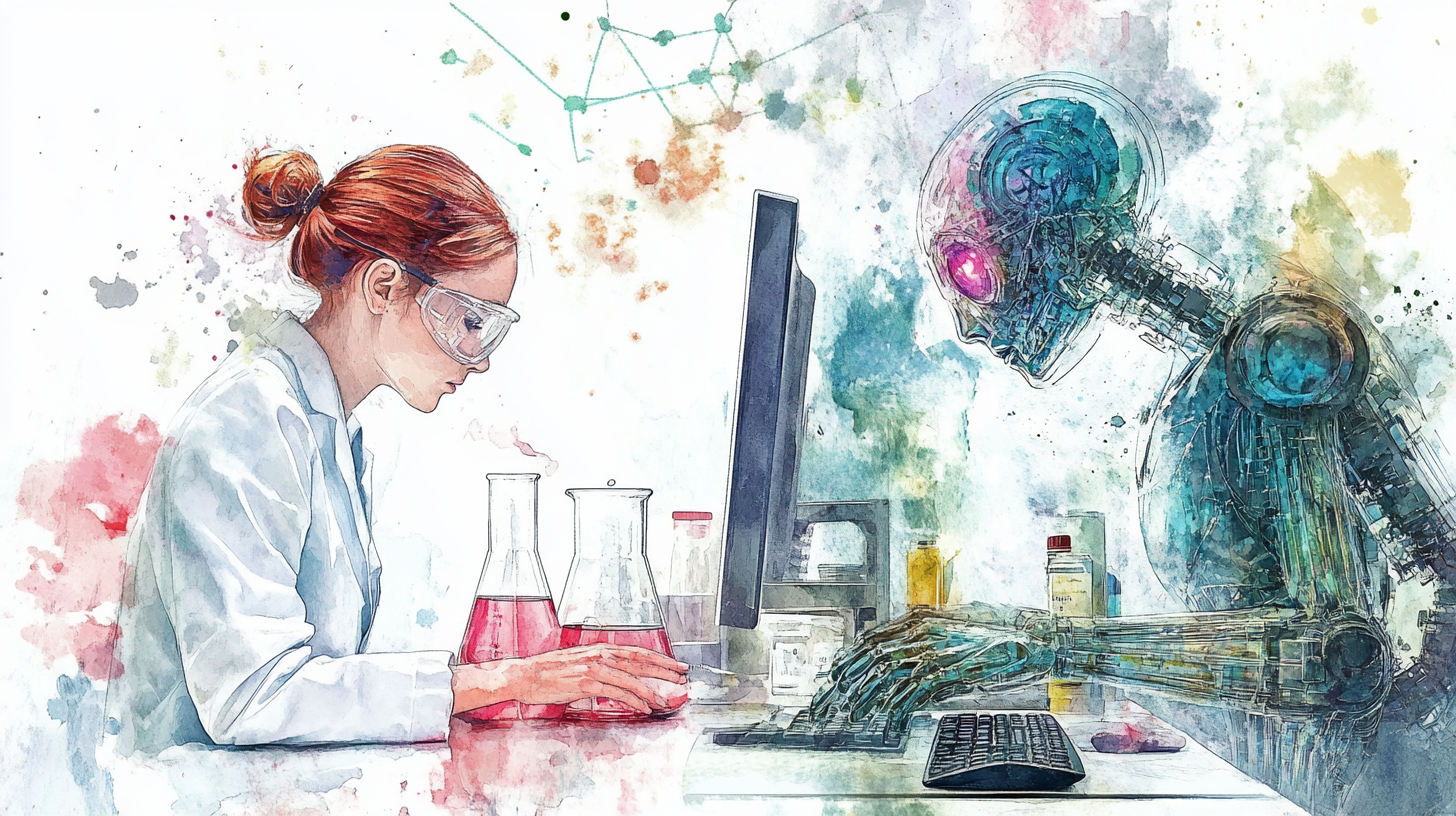
"Are you an AI/ML company?"
This might be the most expensive question in biotech right now. Not because the answer matters, but because we're asking it in the first place.
We recently looked at our most established enterprise partners and noticed something striking: only two identify outright as "AI companies", yet over half are doing sophisticated AI/ML work that's fundamental to their pipeline. This isn't just a quirky observation – it's a reflection of how the biotech industry has arbitrarily divided itself into opposing teams.
On one side, you have the 'traditional' companies: medicinal chemists designing compounds, assay teams running experiments, the classical march from target identification to clinical trials. On the other, you have the 'AI companies': platforms, predictions, algorithms promising to revolutionize drug discovery. This division has spawned two equally destructive camps. The traditionalists point out that there's never been an AI-nominated drug approved. The AI evangelists claim we can predict properties in hours rather than years. Both miss the point entirely.
The reality? Most successful biotechs operate (or will operate) somewhere in the middle. They're not building autonomous AI platforms that will replace scientists and magically predict accurate drug properties in <1 minute, nor are they ignoring the efficiency gains that computational tools can provide. Instead, they're finding practical ways to speed up development: using ML to prioritize which experiments to run first, optimizing assay conditions algorithmically, or predicting protein structures to accelerate design work. We're talking about concrete improvements – X% faster experiment design, Y% more efficient data analysis – not fantastical claims about replacing traditional R&D.
But here's where it gets expensive. When companies feel pressured to pick a side, they make costly decisions. Traditional teams, wary of AI hype, might refuse to build even small data science teams that could dramatically accelerate their existing work. AI-focused companies, eager to differentiate themselves, might over-invest in computational infrastructure at the expense of more foundational wet lab expertise to validate their results. Both scenarios waste time and money.
If you've ever taken a graduate-level course in computational biology or ML, you know that one of the first things you learn is that many of the core mathematical principles underlying computational methods were developed many decades ago. They're fundamentally statistical methods – the same ones taught in stats courses. But today, no one asks whether a biotech company is a 'statistics company'. It's a given that strong statistical expertise is fundamental to good science. Yet somehow, when those same statistical principles are applied at scale through something like machine learning, we act like companies need to pick a side.
To be clear, skepticism in biotech is natural and often healthy. We work in an industry where being wrong can have serious consequences. But there's a difference between healthy skepticism and reflexive resistance to new approaches. Sometimes, innovations that seem too good to be true do have the potential to be transformative.
Take Ozempic. The drug's impact has far exceeded what many initially thought possible. Beyond its primary use in diabetes, clinical data has shown it reduces major cardiovascular events by 26%, drives sustainable weight loss of 15-20%, and shows promising effects on kidney function and fatty liver disease. A single mechanism – GLP-1 receptor agonism – turned out to have such profound, system-wide benefits that we're still uncovering new therapeutic potential years later. If you'd described these benefits decades ago, many people would have been deeply skeptical.
This pattern – initial skepticism followed by data-driven validation – is a recurring theme in biotech. The key is being able to distinguish between healthy scientific skepticism ("show me the data") and reactive resistance ("that can't possibly work"). The best biotech companies we work with know how to toe that line. They don’t wait to resolve the philosophical question of whether they were 'AI companies' before adopting tools that worked. They simply ask: "Will this help us develop better drugs faster?"
The future of biotech isn't about choosing between traditional drug discovery and AI. It's about being smart enough to recognize valuable tools when we see them, while maintaining the healthy skepticism that's core to good science. The companies that will win aren't the ones with the cleanest ideological stance – they're the ones pragmatic enough to take advantage of every possible edge in the race to get drugs to market.
If you want to chat more about anything we wrote, or you’re interested in finding a way to work together, let us know!