Ending the era of data-blind project managers
Project management in bio is a unique challenge of managing a complex web of data, milestones, decisions, and contexts over prolonged periods of time. Traditional PM tools weren't built for this reality – they're straining under the weight of trying to manage work they weren't designed to understand. Here, we explore some reasons why. If anything resonates, please reach out!
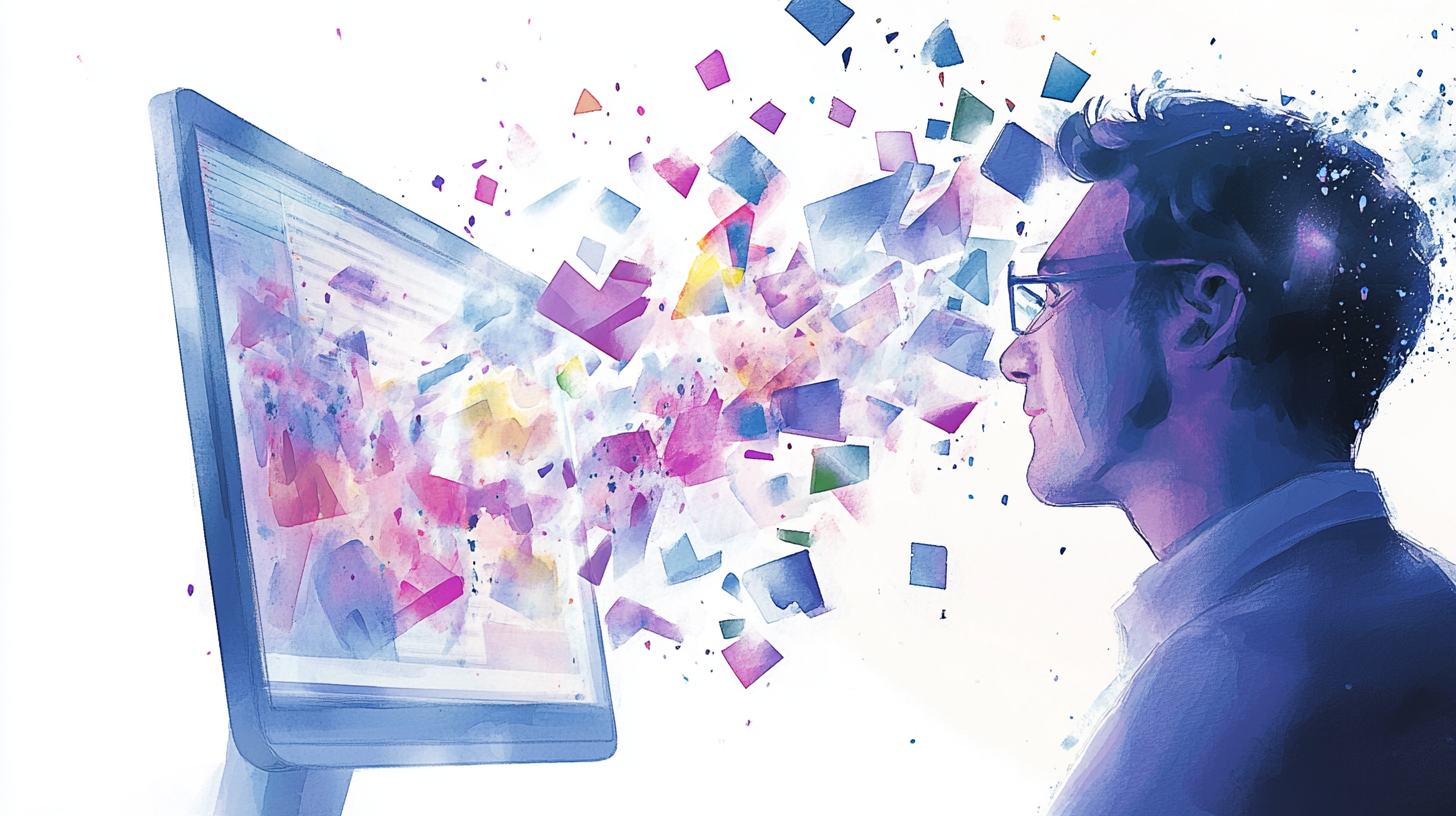
If you work in or around software, you know of the saying: "we shape our tools and they shape us back." This is playing out in Life Sciences… but in all the wrong ways. For decades, Project Managers in bio companies have borrowed traditional PM tools from non-vertical software, and now find themselves contorting their R&D workflows to fit tools that fundamentally don't understand their work.
In traditional project management, work flows linearly: you plan tasks, execute them, and move on. Tasks are discrete units that can be checked off and archived. But in Life Sciences, this entire model breaks down because your tasks often generate data – critical output that drives your next steps. Every experiment generates results that influences what work happens next.
This creates a fundamental disconnect when Life Science teams try to use traditional PM tools. Imagine trying to run a CRM without access to your customer database, using an email client without an address book, or managing your personal finances without connecting to your bank account. You could do it, but you'd be deliberately cutting yourself off from information that should be driving your decisions. Yet this is exactly what happens when PMs at biotechs are forced to manage their work in systems completely disconnected from their experimental data. As a result, teams using platforms like Benchling or CDD Vault end up doing double the work, manually tracking in their project management systems what's already happening in their ELN (plus various other bio tools). This fragmentation introduces massive inefficiencies, increases risks of errors in manual translation, and creates gaps where insights that matter slip through.
The problem compounds when you look at what we're actually tracking. In Life Sciences, the molecules, proteins, sequences we track are complex entities with their own attributes and relationships that matter for planning work. A compound synthesis queue isn't just a series of tasks - it's tracking the flow of actual molecules through multiple stages, where each stage's results shape the next steps. Without tools that understand these relationships, teams spend hours reconstructing context that should be readily available.
The pain really shows up in how R&D actually progresses. Traditional PM tools are built around sprint cycles with clear endpoints, but Life Science projects involve massive numbers of experimental iterations running in various combinations over months or years. You might need to track many-to-many relationships between entities and experiments, follow molecular lineage across generations, or understand how different constituent pieces came together to create a final entity. And this goes way beyond complex dependencies – it's about maintaining a clear understanding of how your scientific work evolves and branches over time.
Consider what happens in a typical week at a biotech company. Your computational team has generated predictions about promising compound variants. Your wet lab is running assays on current candidates. Your CMC team is working on process development. In traditional PM tools, these become isolated task lists. But, in reality, all of these work streams are deeply interconnected, with data flowing between them that influences each team's process. When your tools don't understand these connections, teams waste time in coordination meetings just trying to piece together the current state of their work.
The impact becomes even more apparent in weekly leadership meetings and partner reviews. Instead of focusing on scientific insights and decision-making, teams spend hours preparing updates by manually collating information from different systems. What should be a straightforward review of progress becomes an archaeological dig through multiple platforms, trying to reconstruct the story of what happened and why.
Even the basic assumption about how long work stays relevant falls apart. Traditional PM tools are intentionally ephemeral - once a task is done, you archive it and move on. But in R&D, that experiment you ran two years ago might hold really important insights for your current work. When your historical context matters this much, treating completed work as disposable just doesn't make sense. This becomes especially critical as programs advance and teams need to piece together the full history of their development process.
This challenge is only growing as biotech becomes more data-driven. The volume and complexity of data being generated by modern R&D teams is exponentially increasing. Teams are running parallel experiments across multiple modalities, using sophisticated analytical tools, and generating rich datasets that influence their development decisions. Traditional PM tools weren't built for this reality – they're straining under the weight of trying to manage work they weren't designed to understand.
This is why we built Kaleidoscope to combine data review with work planning. When scientists review their results in our dashboards, they can seamlessly queue up next experiments. We treat compounds as first-class attributes, making it easy to filter and sort experiments based on the compounds present, programs they belong to, and labels that classify assay type. Our experiment types come with structured templates that understand what kind of data they'll produce, making it simple to spin up new experiments and find them later. These templates can include subtasks and sub-experiments, enabling teams to plan complex multi-step processes while maintaining clear connections between work and data.
The implications go beyond efficiency. When your work management truly understands your science, you unlock new possibilities for collaboration and insight. Teams can easily track experiments across different stages and types. Scientists can quickly find historical context for their current work. Leaders can see clear connections between different workstreams. Partners can be kept aligned with real-time visibility into relevant progress. All without the manual overhead of trying to force scientific work into tools that weren't built for it.
Ultimately, in life sciences, your project management is only as good as its connection to your data. We can't keep pretending that tools built for software development will somehow meet the unique demands of R&D. Weekly review sessions shouldn't require jumping between multiple systems. Planning next steps shouldn't mean manually translating your experimental results into a format your PM tool can understand. It's time for solutions that recognize there’s an inseparable connection between scientific work and the data it generates - tools built for how R&D actually works.
If you want to chat more about anything we wrote, or you’re interested in finding a way to work together, let us know!