Plan smartly, execute precisely: the high-stakes game of chasing biotech value inflections
Successfully reaching the market in biotech hinges on building and maintaining a well-defined roadmap. One that clearly outlines the milestones you need to hit, the data to convince you you've hit them, and the experiments that will generate that data. In this Kaleidoscope post, we outline why disciplined, proactive planning is such a critical ingredient to biotech success. If anything here resonates, please reach out!
Developing a new drug means progressing through consecutive milestones to get to commercialization. All of R&D follows a similar lock-step pattern, where reaching each milestone relies on successfully completing the previous one to move forward – like levels in a video game.
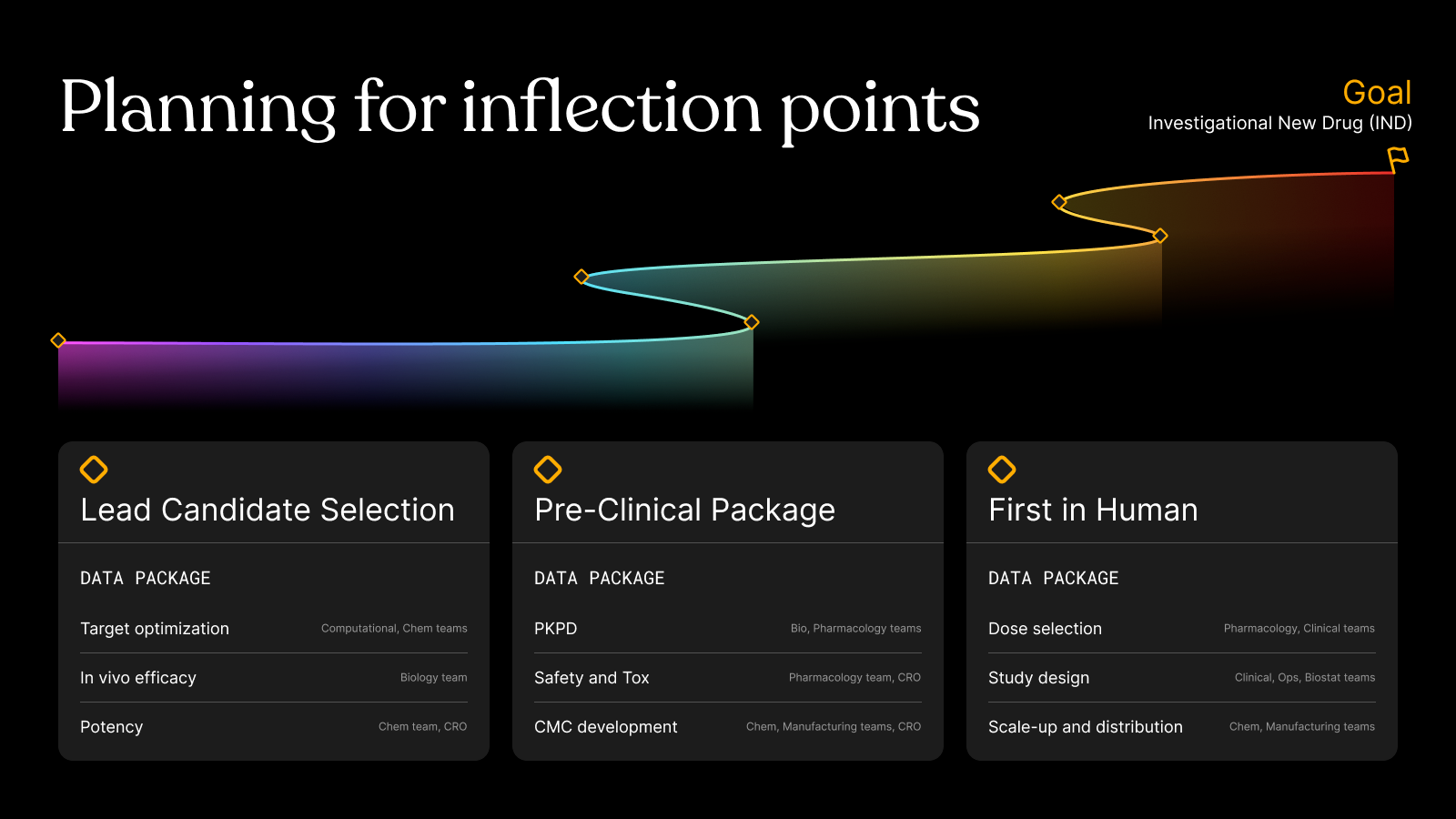
To make it through this video game and get to market, you have to navigate a plethora of inflection points: various funding challenges, preclinical and early clinical milestones (proof of concept, toxicology data, early FDA interactions, IND-filings), first-in-human studies, phases of development (Phase 2 + 3), and commercialization. 90% of clinical drug development never makes it to the end of this process – and often not because of challenges with science, but because of an inability to overcome organizational obstacles that get in the way of good science. And these organizational obstacles usually crop up where there’s a lack of planning.
Simply put, the best biotechs have a clear map of how to navigate each milestone. They understand the data needed at each phase of development and start with the end in mind. By knowing the ultimate goal, biotechs are able to understand the data necessary to support this goal, and can better define the experiments that will generate this essential data. This way, they avoid costly pitfalls and ensure they’re laser-focused on the right activities rather than chasing irrelevant data or duplicating efforts unnecessarily (which further accelerates burn and shortens runway).
Using pre-clinical milestones as an example, it’s easy to inadvertently create pitfalls when identifying preclinical to clinical endpoints. When selecting an animal model, for example, the most important criteria is selecting models that are the strongest mirror for the target disease phenotype – i.e. animal systems that most closely resemble human disease progression and have the capacity to reproduce the disease or pathology as much as possible (yes, animal models have their limitations in how translatable they are, but that’s a topic for another post).
The disease manifestations and timing of the animal model need to be as close as possible to that of a human for you to be able to properly align your target patient group to your animal data when it comes to progressing to the first-in-human stage. As part of your IND submission, you’ll need to finalize all preclinical to clinical translatable endpoints by being able to say: “we’ve seen this progress in the animal for X, we’ll see a similar response in a human for Y.” Ignoring this level of detail going into the preclinical phase is failing to see how this data fits into the bigger picture down the line – especially when ‘down the line’ comes sooner than you think.
A couple of weeks ago, Building Biotechs came out with a great podcast episode with Kyriakos Economides, VP of Translational Pharmacology at Werewolf Therapeutics. When asked when companies should start thinking about their clinical prospects, he answered: “very early.” The preclinical work often happens within the first year of getting meaningful funding and, from there, investors like to see IND filings within two years. So, although you think you might have years to go in the clinical process, you start moving through that process pretty quickly – meaning biotechs need to be able hit the ground running as soon as they have investors on board. For Kyriakos, this means bringing someone in early, whose job it is to think about the full picture of what it takes to get your drug to market (preclinical, in vitro, in vivo, etc.).
The goal is to make each step, each experiment, count towards a larger, strategic objective – and this is much easier to do when you’re data driven. Nicholas Larus-Stone wrote a great thread recently in response to Jesse Johnson’s post on whether biotech is data driven, saying that being data-driven means being deliberate about the decisions you make. He makes a good point: “It should be obvious when data is generated outside of “normal” ranges and easy to figure out whether that’s due to biological variation and technical variation. Tech companies put up huge monitors with important metrics. Why don’t biotech companies do the same?” His answer is that our tools are letting us down and aren’t fit to facilitate the data-driven process biotechs need to survive each milestone. We agree, which is why we built Kaleidoscope to fill a painful gap that we saw many biotechs struggle with.
Kaleidoscope gives teams a framework to map strategic milestones to corresponding data to underlying experiments, and track their progress towards critical business goals. In our last post, we wrote about how well-structured biotech data rooms can catalyze progress through inflection points, like a fundraise or partnership. The same is true of R&D milestones. Having all your key information in one trackable place or dashboard - like headline results from experiments against key milestones and project timelines - makes it easier to see what data is being generated and align your wider team on why that data matters in the first place. It’s a way of ensuring that every experiment is designed not just to test a hypothesis, but to yield data that feeds into a larger goal or effort. David Li shared a similar thought: it's about defining milestones in terms of the data needed, to avoid wandering, unbound experiments.
Having a plan isn’t a one-time thing. It demands continual reassessment and adaptation - qualities that echo the method of 'build, measure, learn.' For biotechs, this could translate to iterative cycles of experiment, data analysis, and refinement of research direction. The old adage of "fail fast, fail cheap" doesn’t quite translate to biotech R&D. Instead, it's more: plan smartly, execute precisely. The stakes are high, and the resources precious. Having a clear map in place to get to the next inflection point is not just about efficiency – it's about survival.
If you want to chat more about anything we wrote, or you’re interested in finding a way to work together, let us know!